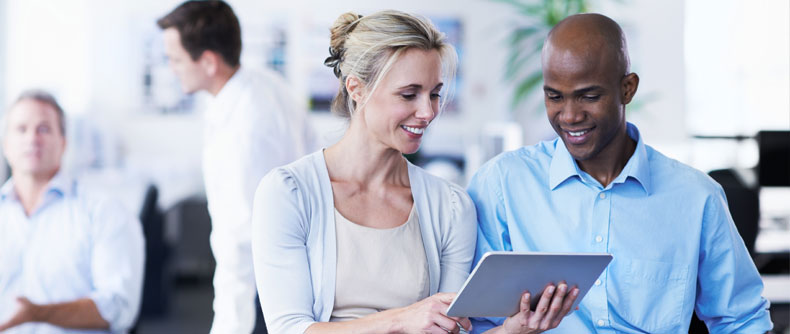
Data-literate companies possess the ability to retrieve, structure, analyze, consume and leverage data to their advantage.
A seemingly infinite number of sources continue to produce endless streams of data that grow deeper, more complicated and granular each day. In banking, this means that fluency in the practices of data analytics and data science is paramount.
As even the smallest variance can cause drastic market shifts, finance professionals of all ilks – from fund managers, analysts, chief investment and financial officers, to wealth management advisors and financial planners – need continuous access to intelligence that is both accurate and timely.
Data-literate companies understand facts and figures, and that there are many stories to be told in petabytes of available data just waiting to be collected from financial records, IPO filings, customer feedback surveys, economic indicators, and consumer trends. By itself, data has scalable value. But with the application of machine learning, automation and artificial intelligence (AI), such as natural language generation (NLG) technology, these records blossom into a cornucopia of valuable insights.
People continuously tout the value of big data – and with good reason. It makes sense to think that having more information should increase the likelihood of decisions that produce positive outcomes. But what gets overlooked is the risk, frequency and impact of bad data.
The sheer volume of information that’s out there, and the speed at which it goes public, is unfathomable. With so many moving parts, mistakes are a matter of “when,” not, “if,” and one reason why erroneous financial reporting is on the rise.
For public companies, automating the intake and analysis of both structured and unstructured data with public filings, and flagging changes in sentiment with a research analyst, can improve the speed and accuracy of financial reporting.
According to the SEC’s annual report, in 2018, financial restatements involving material inaccuracies in a prior year’s financial statement increased for the first time since 2006. Also, a recent MarketWatch analysis of 100 IPOs SEC filings in 2019 showed that a number of those companies later disclosed serious issues with internal controls over accounting, financial reporting and the systems.
Bad data is a real problem – this cannot be overstated.
Results from a 2018 survey of 1,100 C-level executives and finance professionals in midsize and large organizations validate the cause for concern. According to Censuswide, the research firm that conducted the study:
• 69 percent of respondents said that their CEO or CFO has made a significant business decision based on out-of-date or incorrect financial data, while;
• 40 percent attributed their lack of confidence in the accuracy of their financial figures to being overwhelmed by so many data sources.
These results paint a frightening picture: a majority of FP&A personnel knowingly create financial reports that present findings based on data that is either outdated or inaccurate, indicating widespread weaknesses in internal controls over financial reporting (ICFR).
Failing to address ICFR deficiencies creates a reasonable possibility that a material misstatement of the company’s financial statements — whether due to error or fraud — will not be prevented or detected on a timely basis, reported MarketWatch. It doesn’t help matters that manual data entry and related activities are time-consuming and tedious.
While it may not matter to employees of Initech (Office Space) if the cover pages of TPS reports are missing, in the real world, simple transposition, calculation and other original entry errors that go unnoticed can land companies in the crosshairs of SEC auditors.
Automating repetitive manual processes helps maintain data integrity, removing the risk of mistakes with data entry or other manmade errors that skew findings derived from datasets. Tainted data points business leaders in the wrong direction, causing them to make decisions based on the wrong information.
Robotic process automation (RPA) platforms instantly and automatically collect and organize data from disparate sources into structured formats, drastically reducing the potential for human errors. According to Forrester, RPA robots are capable of mimicking many–if not most–human user actions. They log into applications, move files and folders, copy and paste data, fill in forms, extract structured and semi-structured data from documents, scrape browsers, and more.
Considered the last mile of data, NLG enables the analysis, assessment and communication of data with precision, accuracy, and scale, allowing people to focus on more creative, high-value activities. Most RPA platforms present analyses in the form of data visualizations. Natural language narratives augment data visualizations, identifying new trends and hidden metrics.
NLG adds contextual commentary and drills down analysis that complements illustrative graphs and charts, and which are indistinguishable from analyses written by a human subject matter expert.
By mining structured datasets, NLG transforms non-language statistical data in Excel spreadsheets, metadata, videos, and other datasets into legible (or audible), natural-sounding narratives.
From shareholder reports to individual client financial portfolio summaries, NLG furthers your data’s ability to tell a story. And with timely access to insights, executives are empowered to make better, more informed business decisions, faster.
Artificial Intelligence & The Evolution of Natural Language Generation
As I stated in the beginning of this article, data literacy is paramount. The ability to manipulate and analyze vast quantities of both structured and unstructured data can help uncover trends and produce actionable insights that may otherwise be lost in the vortex that is “Big Data.”
Today, artificial intelligence vis-a-vis natural language generation technology fuels operations across the spectrum of financial institutions functions, including: customer experience; asset management; compliance; shareholder communications; financial reporting; fund analysis; and more.
Business intelligence dashboards, like Tableau and Microsoft Power BI, produce analysis of structured data as data visualizations and tabulated reports. Over time, the desire to complement these data visualizations with distinctively human narratives has grown, spurring the adoption of natural language generation.
NLG adds the ‘Power of Language’ to the presentation layer of business intelligence in to help convey the meaning and implications of underlying data.
Sophisticated NLG platforms can delve into large quantities of numerical data, identify patterns and translate the obtained information into natural-sounding words and sentences. These capabilities are highly applicable to corporate reporting.
NLG instantly converts structured data sets from multiple streams into fluid contextual analysis that’s delivered in everyday vernacular, adding explanatory storylines that extend the value of data visualizations and automation.
Timely access to insight is a distinct competitive advantage for executive leaders who can identify and take advantage of new market opportunities, about which their data-illiterate competitors are not aware.
Enterprises can no longer afford to ignore the impact of automation and artificial intelligence. With new use-cases being born each day, the stage is set for even greater disruption of business processes and activities.