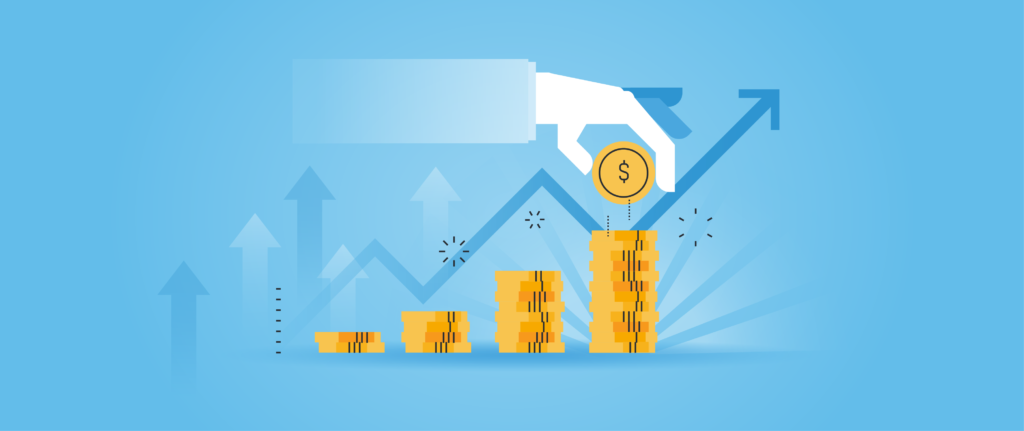
As the banking, financial services, and insurance industry continue to navigate and address the challenges of scale, efficiency, and innovation, here are seven of the most common use cases for natural language generation (NLG), the engine of Arria Investment Analyst.
Our offerings allow customers the choice to select from an appropriate combination of Reporting as a Service (via RESTful API), URL Web platform, Dashboard Plug-ins with the major BI players (MS Power BI, Tableau, MicroStrategy, Qlik, and TIBCO), and with MS Excel.
Investment performance commentary
There is an obvious natural fit to ease the burden and some of the friction points of the reporting value chain. Very often the value chain process can only be sequential and spans multiple business unit departments. All the inputs and vendors, by definition, must-have components with common data dimensions (hierarchy-classification fields/buckets/weights/returns/attribution effects/ holdings/transactions) and measures (time periods, top/bottom holdings, reporting configurations/drill-thru capabilities). It is no longer the case that skilled humans need to replicate the same process repeatedly. Any data issue or change often needs to reset the value chain, perhaps even from the beginning accounting book of record. Our technology eases the strain, is scalable, and will not make human mistakes. This in turn allows skilled humans to add value (stock stories, market outlooks, conviction plays, customization to the voice of the firm) and not spend time on low-value (but factually critical) elements.
Intent/categorization/P&L drivers
Retail banking apps and platforms very often solely produce a value over a defined or static period and some limited or proprietary categorizations of the data. There is normally no ability to customize or see thematic trends or any-date-to-any-date time periods nor, dynamically on the fly, combine periods and categories together via the application/presentation layer interface. These user problems can be solved with simple clicks of navigation buttons with ARRIA.
Environmental Social Governance (ESG)
With over 150 vendor market providers of ESG, increasing regulatory pathways, and industry bodies bringing in examinations/certificates with a view to nurturing consensus of approach, we find companies and vendors are developing ideas to provide common taxonomy. There is a long way to go. To our awareness, there is currently only one published ESG attribution methodology. This allows artificial intelligence to explain the drivers of the investment process with an ESG lens, in addition to the traditional attribution factors.
Ex-post risk **
The most used ex-post risk statistics include tracking error, information ratio, Sharpe ratio, standard deviation, (Jensen’s) alpha, and beta. Often these mathematical statistics are calculated outside of the main investment performance return engines or in a separate module. A business intelligence tool or reporting package is needed to surface returns and attribution with ex-post risk statistics (and certainly ex-ante risk data). Our capability includes plug-and-play connection templates allowing fast integrations, measured in days. Gone are the days of twelve-to-eighteen-month implementations.
Credit risk analysis/research
The analysis and research often span multiple sources and mediums (depending on the intention/asset type) but always comes back to a standard schema determined by the firm or market convention. A combination of Robotic Process Automation and NLG enables screen-scrapping and curated analysis to be implemented and scaled. Between 20%-40% of an entire workflow process can be removed from the operating model. Simply use the time gained more wisely with your skilled workforce.
Broker analysis
The market never sleeps. Brokers need to surface their recommendations as soon as practically possible when results or a market event occurs. In the past, humans have been required to scale. A computer never gets tired and processing power is cheap. Artificial intelligence can repeat processes continually to surface insights in real-time.
Stress testing scenario analysis/ex-ante *** /construction
An extension of the issue is covered with ex-post risk statistics. The dominant providers in this space are not the exact dominant providers of the portfolio and or benchmark returns nor the investment performance attribution. Very few have seamless integration and reporting capability. Fewer vendors still offer portfolio construction and stress testing scenarios on the same data sets (Accounting book of records e.g., custodians) as the client statements, and most use the Investment Book of Records (e.g., in the trading platforms). However, again the market identifiers (ISIN, CUSIP, SEDOL) and the holdings information are consistent across these platforms.
ARRIA Investment Analyst offers compelling reasons to explore these use cases in your organization.
To learn more, see it in action.
________________________
Endnotes:
*Journal of Performance Measurement, Measuring the Contributions of SRI/ESG Investment Strategies
Philippe Grégoire, Ph.D.
Source: Investopedia
**What Is Ex-Post Risk?
The term ex-post risk refers to a risk measurement technique that uses historic returns to predict future risks associated with an investment. This type of risk manages risks associated with investment returns after the fact. Future risk is determined using the statistical variance from the relative mean of long-term returns in the past for a particular asset.
***What Is Ex-Ante Risk?
Ex-ante risk refers to any of the returns that an investment earns before that risk actually takes place.
This kind of analysis looks at the risk of current portfolio holdings and estimates future return streams and their projected variability based upon statistical assumptions. An example of ex-ante analysis is when an investment company values a stock ex-ante and then compares the predicted results with the actual movement of the stock’s price.
To our awareness, there is currently only one published ESG attribution methodology*.