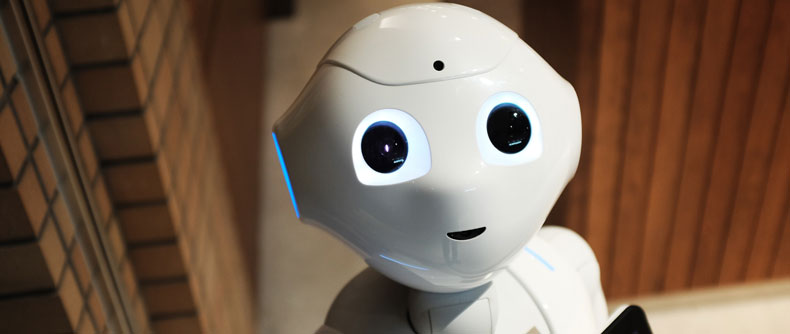
I am very excited about the potential of using chatbots to explain data to people. So far, chatbots have mostly been used for simple transactions like ordering pizzas, but they can do much more than this! After all, if one person wants to communicate insights to another, they’ll probably do this via a dialogue (chat). Why shouldn’t computer insight-presentation tools work the same way? Gartner has identified “Conversational Chatbots for Analytics” as a new and rising element in their Hype Cycle for Analytics and Business Intelligence.
I see the need for NLG analytics chatbots in my university work as well as at Arria. For example, one of my PhD students has developed an app which gives people feedback and insights about their diet. When he asked people to use the app, one of the most common reactions was that people wanted to ask it questions. For example, if the app suggested that they eat more protein, they might want to know why protein consumption is important, how much protein they should eat, what foods in their current diet were providing a lot of protein, how they could change their diet to eat more protein, etc. If my student had included all of this information in his report, it would have been very long, and most people probably would not have read it. Far better to have short summary commentary with key insights (such as “eat more protein”), supplemented with a chatbot so that people could ask detailed questions about things they care about in a more interactive, dynamic way.
Of course, achieving this vision requires a lot of hard work to develop the appropriate technologies for use in chatbots — NLU (natural language understanding) to understand what the user says, and NLG (natural language generation) to produce a good reply. From an NLG perspective, there are challenges with chatbots that don’t occur with report generation. For example:
- Chatbots often need to add extra information to their response so the user can check that her question was correctly interpreted. Thus, if the user asks, “What were sales in France in January”, the chatbot might reply “In January 2020, sales in France were $150,000”. In this case, the user specified the month but not the year, so the chatbot added the default year (2020) to the response; if the user meant a different year, she can ask the question again with a year specified.
- Good chatbots also add extra information when a literal response is misleading. For example, if the user asks “Which country had the highest sales”, and sales in France were $150K while sales in Canada were only slightly lower at $146K, then it is probably better to respond with “France and Canada” instead of just “France”.
- If narratives are spoken, then the chatbot should avoid repeating currencies. For example, “Sales rose from $1M to $2M” is verbalized as “Sales rose from one million dollars to two million” (i.e., we say “two million” instead of “two million dollars”).
There are many other such examples. Most people who use chatbots don’t even notice such things if the chatbot gets it right; but they will notice and complain if the chatbot gets it wrong!
Arria has just announced the first version of its analytics chatbot, Arria Answers, which handles NLG issues such as the ones I mentioned above. We hope you are as excited as we are about the potential of analytics chatbots!